Table of Content
Build an ETL pipeline for extracting IoT sensor data from assets, transform it into useful information, and load it into data lakes or a data warehouse.
Data comes at high velocity from different sources, requiring companies to produce real-time insights for business decisions. Data streaming helps to process data in real time (velocity) with low latency, in seconds or minutes, and to react to data almost immediately (response analytics).
Streaming ETL starts with the acquisition of data from IoT devices. The data is then transformed into a useful format in order to process it in a centralized location (data lake). Streaming ETL pipelines help to ingest efficiently, process, and analyze larger volumes of data continuously to reach decision makers faster.
Architecture for etl pipeline
Data Source Extraction – Ingestion – Storage – Processing – Destination
An ETL pipeline sources data generated by connected IoT devices, sensors, and equipment, ingesting it into cloud storage (separating resources and ingestion mechanisms), making it accessible in real time to processing solutions (ETL and analytics), and then sending it either to warehousing and data lakes or directly to other applications to respond with alerting, notifications, emails, etc.
Data streams can be handled using open-source tools and cloud storage services to capture and store data, analyze it in real time, and perform ETL processing for machine learning, marketing data integration, or the movement of data to other destinations such as Amazon S3 or Google Cloud Storage using API calls.
Data streams require setting up configurations to clean and organize data and prepare it to be converted and consolidated into larger files (compression, encryption, and other processes) before it can be connected with tools. Next, data is cleaned and made available to be processed by analytical tools.
IoT devices and sensors connect with IoT platforms through MQTT endpoints, which route event streams to other services through APIs, set up to ingest data, and provide durable storage and streaming solutions with minimum code.
IoT cloud data integration platforms offer many prebuilt connectors and transformations to connect to data cloud services and allow ETL pipeline portability. In addition, the storage and processing stage allows data to be set up for batch processing and adds many functions adapted to the use case scenario.
The ETL processing and streaming process helps clean up data and transforms it with format conversions, data buffering, compression, etc., before it arrives at your data lake and connects with other applications.
Other more complex approaches may require more code but may also offer more flexibility, such as ingesting data using virtual machines, Kubernetes, and other elastic tools that support hybrid and multi-cloud strategies (hybrid enablement) and work together with other data streaming processing solutions to reduce batch processing and latency.
Data ingestion receives data in batches, micro-batches, or constant streams, depending on the use case, timing needs, connectivity, latency requirements, costs, etc. Thus, data is processed in batches or streams, or by both methods.
Data is ordered, sorted, and grouped, with many elements flowing cohesively before it is sent to processors. ETL scripts are written to create functions that trigger the processing of data. Batch processing is usually more efficient and less costly than streaming processing.
- Stream Processors handle data in continuous streams.
- Batch Processors handle data in aggregate batches.
Data comes in binary format. Sensor data streams are decoded before processing (stateless operation), so it is important to discuss how to handle and group data, create checkpoints, and manage other issues related to these ETL pipeline processes.
ETL frameworks have features that can help manage states, cluster checkpoints, decoding, stateful operators, synchronization, etc.
Once data is processed and loaded to data lakes, warehouses, stores, or targeted databases, it is ready to be used for computing data products and other applications.
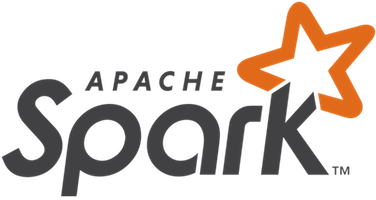
ETL with Spark
You can build an ETL pipeline with Apache Spark (parallel processing framework) to perform streaming, batch processing, or data querying before loading to a data lake. ETL with Spark allows aggregating data effectively from many sources and supports multiple programming languages. Public cloud services have Spark connectors to implement Apache Spark in the cloud and computing systems such as Kubernetes and Hadoop. You can interact with Spark through Google Dataproc to run ETL jobs and create and update clusters.
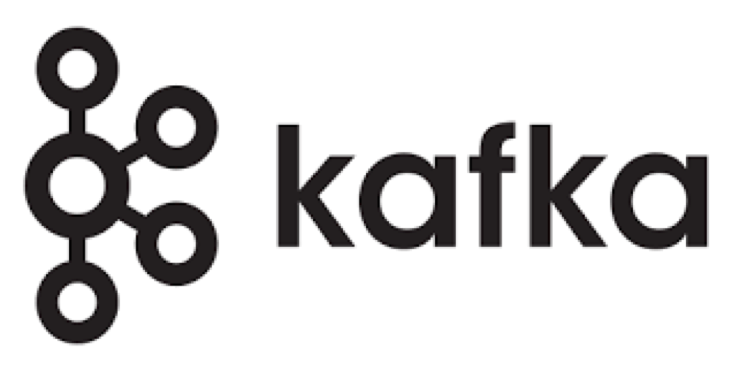
ETL Kafka
Apache Kafka is an open-source distributed event streaming platform for high-performance ETL pipelines. It provides connectors that work with its own framework tool (Kafka Connect) to stream data (import/export) between Kafka and other data systems. Kafka extracts data from many different sources, performs transformations within applications, and loads data to other systems. Kafka has client libraries (Kafka Streams APIs) for building apps and microservices that store data in clusters and create complex transformations with a flexible ETL architecture and real-time capabilities.